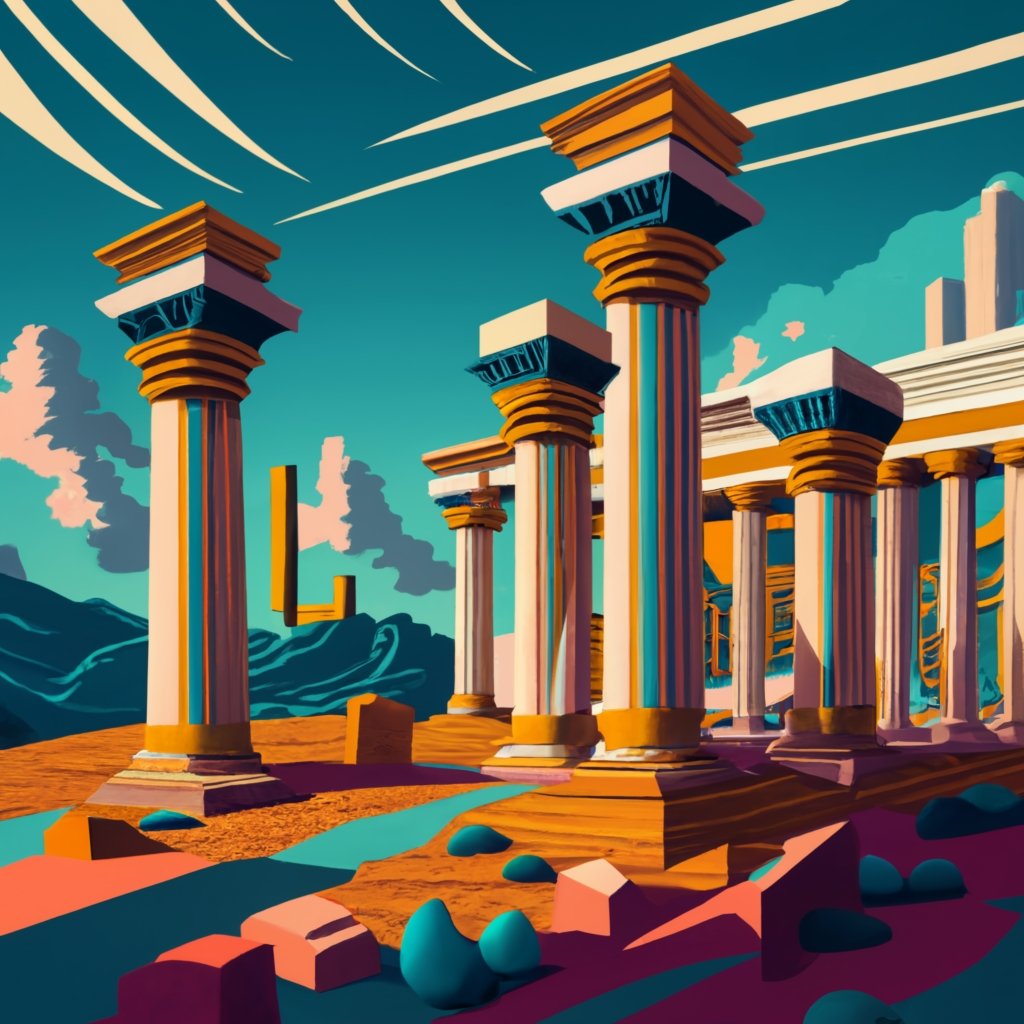
The 5 Pillars of Data Quality: Building a Strong Foundation for Informed Decision-Making
In today’s data-driven world, businesses and organizations rely heavily on data to make informed decisions, gain insights, and stay competitive. However, not all data is created equal. Ensuring that your data is of high quality is paramount to deriving meaningful and actionable insights. This is where the 5 pillars of data quality come into play: accuracy, consistency, completeness, reliability, and relevance. Let’s explore each of these pillars and understand their significance in maintaining data quality.
1. Accuracy
Accuracy is perhaps the most fundamental pillar of data quality. It refers to how well the data reflects the real-world entities or events it is meant to represent. Inaccurate data can lead to costly mistakes and misguided decisions. To ensure accuracy, data should be collected with precision and free from errors. Regular data validation and cleansing processes should be in place to identify and rectify inaccuracies.
2. Consistency
Consistency ensures that data remains uniform and coherent across various sources and over time. Inconsistent data can create confusion and undermine trust in the information. Data consistency involves using standardized formats, units, and naming conventions. Data integration and data governance practices play a crucial role in maintaining consistency.
3. Completeness
Completeness assesses whether all the required data elements are present. Incomplete data can result in gaps in your analysis, leading to inaccurate conclusions. To achieve completeness, data collection processes should be designed to capture all necessary information, and data quality checks should be conducted to identify missing values.
4. Reliability
Reliability is a measure of how dependable the data is for making decisions. Reliable data can be trusted to be consistent and accurate. It is essential to establish data sources and collection methods that are reliable and well-documented. Regular audits and quality assurance procedures can help ensure data reliability.
5. Relevance
Relevance focuses on whether the data is applicable to the task at hand. Irrelevant data can clutter your databases and complicate decision-making processes. To maintain relevance, it’s crucial to regularly review and assess the data you collect, ensuring that it aligns with your organization’s goals and objectives.
Conclusion
In summary, the 5 pillars of data quality are essential for organizations looking to harness the power of data effectively. Neglecting any of these pillars can lead to costly errors, flawed insights, and missed opportunities. By prioritizing accuracy, consistency, completeness, reliability, and relevance, businesses can build a strong foundation for data-driven decision-making and gain a competitive edge in today’s data-centric landscape.